Mini Workshop “Neuroscience and Machine Learning for Advanced Intelligence”
Thanks to deep learning inspired by neuroscience, AI technologies have made remarkable progress recently. The RIKEN Center for Advanced Intelligence Project (AIP) is working for machine learning technologies beyond deep learning, as well as applications of AI technologies to various real-world problems. In order to achieve this goal, it is particularly important to encourage communications and to promote cooperation between researchers in the fields of neuroscience and machine learning. In this mini-workshop co-hosted by RIKEN AIP and the Innovative Research Area “Correspondence and Fusion of Artificial Intelligence and Brain Science” (Organizer: Prof. Doya), we will share research achievements of the latest computational neuroscience and artificial intelligence technologies and discuss how to develop advanced intelligence by learning from the neuroscience.
This workshop is supported by AMED under Grant Number JP18dm0307009.
< Date and Time >
2018.11.13 (Tue) 14:00 – 16:50
14:00 – 14:40 Leonardo L. Gollo (QIMR Berghofer Medical Research Institute, Australia)
14:40 – 15:05 Hidetoshi Shimodaira (Kyoto University / RIKEN AIP)
15:05 – 15:20 break
15:20 – 16:00 Jorge Riera (Florida International University, USA)
16:00 – 16:25 Motoaki Kawanabe (RIKEN AIP / ATR)
16:25 – 16:50 Okito Yamashita (RIKEN AIP / ATR)
< Venue >
Main Conference Room, GF,
Advanced Telecommunications Research Institute International (ATR)2-2-2 Hikari-dai, Seika-cho, Soraku-gun, Kyoto 619-0288 Japan
14:00 – 14:40 Dr. Leonardo L. Gollo (Research Fellow, QIMR Berghofer Medical Research Institute, Australia)
Title: Topological complexity in the brain: Integration, fragility, volatility, and a hierarchy of timescales
Abstract:
The next era of connectomics will be driven by studies that consider both spatial and temporal components of brain organization simultaneously—elucidating their interactions and dependencies. Utilizing this guiding principle, we explored the origin of a hierarchy of timescales in the brain. This is a fundamental link between brain structure and dynamics that has slowly fluctuating patterns of synchronization within the topological core of hub regions, and fast fluctuating patterns at the periphery. We find that a constellation of densely interconnected regions plays a central role in promoting a stable dynamical core (Gollo et al., 2015). This core is crucial for integration of activity from specialized and segregated areas, and it mostly operates in slow timescales. The slow timescales are well matched to the regulation of internal visceral states, corresponding to the somatic correlates of mood and anxiety. In contrast, ‘feeder’ regions surrounding this stable core show unstable, rapidly fluctuating dynamics likely to be crucial for fast perceptual processes. Another implication of this structure-dynamics relationship is revealed by studying the changes in brain dynamics following local stimulation. We find that the same stimulation protocol may cause opposite effects in functional connectivity if the target regions are at the core or at the periphery of the network (Cocchi et al., 2016). These contrary effects are part of a continuous tuning curve that represents how different brain regions respond to stimulation depending on their hierarchical position (Gollo et al., 2017). We also study the fragility and volatility of hub regions to random variants of the human connectome that introduce subtle perturbations to network topology while preserving the geometrical embedding and the wiring length of the brain. If the variation in structure is permitted to accumulate, strong peripheral connections progressively connect to central nodes and hubs shift toward the middle of the brain. Intriguingly, the fragility of hubs to disconnections shows a significant association with the acceleration of gray-matter loss in schizophrenia (Gollo et al., 2018). Finally, we discuss potential applications of brain hierarchy and its integrating core to artificial intelligence and beyond.
14:40 – 15:05 Dr. Hidetoshi Shimodaira (Professor, Kyoto University / Team Leader, RIKEN AIP)
Title: Learning features for relationships with shifted inner product similarity
Abstract:
We propose shifted inner product similarity (SIPS), which is a novel yet very simple extension of the ordinary inner-product similarity (IPS) for neural-network based similarity learning such as siamese neural networks. By combining Mercer’s theorem and the universal approximation theorem, it has been proven that IPS can learn any positive definite similarities. We show that SIPS can learn a wider class, called conditionally positive definite similarities with many examples such as cosine similarity, negative Poincare distance and negative Wasserstein distance. The approximation error rate is also evaluated theoretically. Numerical examples are shown for graph embedding tasks. This is a joint work with Akifumi Okuno (Kyoto University / RIKEN AIP).
15:20 – 16:00 Dr. Jorge Riera (Associate Professor, Florida International University, USA)
Title: A multi-scale approach to evaluate performance monitoring in agranular cortex
Abstract:
Visual inputs into a cortical area change the excitability levels of populations of pyramidal cell (PC), a response that is eventually counteracted by intra-laminar inhibitory feedback from highly-heterogeneous populations of GABAergic interneurons. As a consequence of back-propagating action potentials, changes in PC spiking rates induce fluctuations in calcium activity in apical dendrites, a mechanism that helps these cells get control of their susceptibility to excitatory L2/3 inputs from higher cortical regions (Larkum et al., 1999). Through the action of the hyperpolarization-activated cation current (Ih) (Berger et al., 2003), such an induced gain of PC apical dendrites could be regulated by inhibitory inputs into the PC apical dendrites from Martinotti cells (MC) in the L5/6 cortex (Silberberg and Markram, 2007). This generic multilayer principle for autonomic regulation of PC dendritic gain may underlie important features of cortical processing; therefore, a better understanding might help develop more complex intelligent systems.
In this study, we proposed a two-layer stochastic neuronal mass model to describe dynamic gain control by the dendrites of PCs in the supplementary eye field (SEF), an agranular cortex contributing to an event-related potential (i.e., error-related negativity, ERN) observed in a saccade countermanding task. The model includes populations of PCs with back-propagation induced calcium responses and MC-triggered Ih current control. Combining our local linearization (LL) filter and innovation method, we fitted this model to intracranial (spike-rate/local-field-potentials) and scalp electrical potentials obtained from monkeys performing the task. Finally, we use our model and methodology to: a) predict neuronal activity in the anterior cingulate cortex (ACC), a brain area also contributing to ERN; and b) evaluate potential mechanisms for the genesis of theta bursting observed in conflict processing.
This is a joint work with Arash Moshforoush, Beatriz Herrera, Pedro Valdes-Hernandez, Amirsaman Sajad, Geoffrey Woodman, Jeffrey Schall.
Supported by R01-EY019882, P30-EY008126
16:00 – 16:25 Dr. Motoaki Kawanabe (Department head, ATR / Team Leader, RIKEN AIP)
Title : A machine learning approach to estimate fMRI resting-state network activities from EEG
Abstract:
Recent fMRI research has revealed that a number of networks are consistently found in human brain during resting state. Furthermore, there have been studies reporting relations between such functional organization and personal traits / mental disorders. In order to advance EEG brain machine interface (BMI) for mental state monitoring by incorporating such knowledge in human neuroimaging research, we are developing a novel probabilistic framework called “Stacked Pooling and Linear Combination Estimation” (SPLICE) for a hierarchical extension of independent component analysis (ICA) [Hirayama et al., 2017]. Unlike related previous models, our generative model is fully tractable: both likelihood and the posterior estimates of latent variables can readily be computed with analytic simple formulae. Experiments on EEG demonstrate the validity of the method and showed potential for estimating fMRI resting-state network activities from EEG.
This is a joint work with Jun-ichiro Hirayama (RIKEN AIP/ATR) and Aapo Hyvärinen (UCL/U. Helsinki/RIKEN AIP).
16:25 – 16:50 Dr. Okito Yamashita (Department head, ATR / Team Leader, RIKEN AIP)
Title : Multi-modal integration approach to understand event-related dynamics of human brain
Abstract:
Recent human neuroimaging studies using resting-state fMRI and diffusion MRI have revealed macro-scale network organization of human brain such as existence of functional subnetwork (default mode, attention, sensory and motor systems), small worldness and individual difference of functional connectome. However investigating dynamics on the brain network is challenging partially because of lack of measurement methodology. To address this issue, we have devloped fMRI-informed MEG/EEG source reconstruction method to visualize electrical brain activities in milli-second temporal resolution (Sato et al. 2004, NeuroImage, MATLAB toolbox available from https://vbmeg.atr.jp/?lang=en). Then VBMEG was extended to model and identify network dynamics of electrical activities using connectome (whole-brain structural connectivity) as a building block (Fukushima et al, 2015, NeuroImage). We have proposed algorithm to infer the electrical activities and the dynamics parameters from MEG and fMRI data. Based on the estimated brain network dynamics, we are now developing a method to visualize electrical activities on structural connections which may be useful for understanding signal transmission between brain regions. In this talk I summarize a series of our studies to understand event-related brain dynamics using multi-modal integration approach.
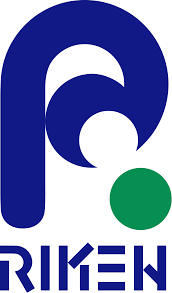
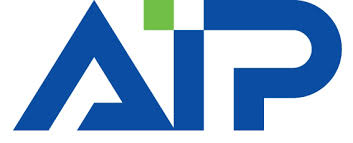
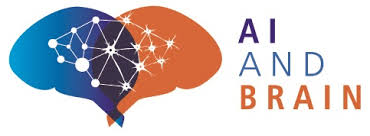
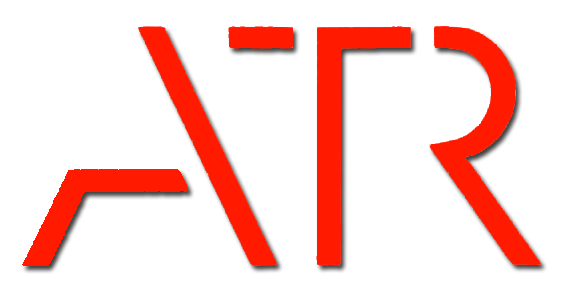
< Co-host >
- The RIKEN Center for Advanced Intelligence Project (AIP)
- JSPS Grant-in-Aid for Scientific Research on Innovative Areas “Correspondence and Fusion of Artificial Intelligence and Brain Science”
< Co-sponsor >